A State of Fear: How the UK government weaponised fear during the Covid-19 pandemic by Laura Dodsworth (feel good novels .TXT) 📕
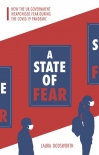
Read free book «A State of Fear: How the UK government weaponised fear during the Covid-19 pandemic by Laura Dodsworth (feel good novels .TXT) 📕» - read online or download for free at americanlibrarybooks.com
- Author: Laura Dodsworth
Read book online «A State of Fear: How the UK government weaponised fear during the Covid-19 pandemic by Laura Dodsworth (feel good novels .TXT) 📕». Author - Laura Dodsworth
The use of universal lockdowns in the event of a new virus has no precedent. Why haven’t they been used before? They are blunt and brutal tools. They breach human rights. (See Chapter 15, ‘Tyranny’.) They are destructive to individuals’ lives and jobs, to businesses and the economy, which ultimately impacts the health of the nation. There was no good evidence that they do work, and in a world which till now has valued evidence-based interventions, that used to be an extremely important consideration. Since the mass quarantining of healthy individuals from 2020, there is a growing body of evidence that this extraordinary measure does not work in achieving its goals.
After a year of global lockdowns, assumptions behind the modelling – that they successfully control the virus and without them hundreds of thousands more would die – have become accepted truth. This ‘truth’ is close to religious dogma here in the UK, where we have endured one of the strictest lockdowns in the world, according to an analysis by Oxford University’s Blavatnik School of Government.1 Only two governments, Venezuela and Lebanon, have introduced tougher policies. Questioning the efficacy of lockdowns is akin to heresy and might earn you the accusatory title ‘Covid denier’ or ‘covidiot’. Have the UK’s restrictions created a population with Stockholm syndrome?
A 2019 report2 Non-pharmaceutical public health measures for mitigating the risk and impact of epidemic and pandemic influenza by the World Health Organization (WHO) warned of the flimsy empirical basis for epidemiology models such as the one developed by Imperial College London. ‘Simulation models provide a weak level of evidence,’ the report noted, and lacked randomised controlled trials to test their assumptions.
The report is about preparedness for influenza, but there would be much similarity in strategies and effectiveness for coronavirus (this was touched upon on in Chapter 1, ‘Fright Night’, and is referenced further in the endnotes). It said that non-pharmaceutical interventions (which include hand hygiene, respiratory etiquette, face masks for symptomatic individuals, surface and object cleaning, increased ventilation, isolation of sick individuals and travel advice) can be effective: ‘by reducing transmission in the community, the epidemic may be spread out over a longer period, with a reduced epidemic peak. This can be particularly important if the health system has limited resources or capacity (e.g. in terms of hospital beds and ventilators). Also, overall morbidity and mortality can be reduced even if the total number of infections across the epidemic is not reduced.’
Isolation of ‘sick individuals’ is ‘recommended’ although the evidence is ‘very low’. Note that these are ‘sick’ individuals. But ‘quarantine of exposed individuals’ is ‘not recommended in any circumstances’. Mass quarantine measures – lockdowns – are ‘not recommended’ due to lack of evidence for their effectiveness. Similarly, evidence for closing schools, contact tracing, avoiding crowding, internal travel restrictions, border exit and entry screening is ‘very low’.
‘Case isolation’ is defined as ‘separation or restriction of movement of ill persons with an infectious disease at home or in a health care facility, to prevent transmission to others’ [italics my emphasis]. The Covid epidemic is the first time that cases have been accepted to be positive PCR or LFT results without a clinical diagnosis. Normally to qualify as a ‘case’, you must be unwell and have symptoms associated with the disease. In this report, even case isolation has a ‘very low overall quality of evidence’, and that’s for people who are sick, not just in receipt of a positive test result.
In summarising the section on isolation, this report, which included the same 2006 influenza model that Ferguson adapted to Covid-19, concluded: ‘Most currently available studies on the effectiveness of isolation are simulation studies, which have a low strength of evidence.’
(Incidentally, nowhere in the chapter ‘Communication for behavioural impact’, in the same report, is the use of fear to encourage adherence to NPIs recommended.)
It cannot be stressed enough that the WHO and the UK have never included mass quarantining of healthy people – lockdowns – in epidemic or pandemic preparedness planning. There was no evidence that lockdowns would work, and the harms were acknowledged to outweigh the potential and unproven effects.
Why did we lock down in the UK? SAGE observed the ‘innovative intervention’ out of China, but they initially presumed it would not be an acceptable option in the UK, a liberal Western democracy. In an astonishing interview for The Sunday Times, Professor Neil Ferguson of Imperial College London, a member of SAGE, said, ‘It’s a communist one party state, we said. We couldn’t get away with it in Europe, we thought… and then Italy did it. And we realised we could.’3
Neil Ferguson’s Imperial simulation model was described in an article in The Telegraph as ‘the most devastating software mistake of all time’.4 The modelling used outdated code and contained multiple flaws. (There is more on the modelling in Chapter 10, ‘The metrics of fear’.)
The doom-laden modelling grabbed headlines around the world and is credited with some of the responsibility for shifting policies on lockdown. And in circular and fallacious reasoning, the success of lockdown in the UK is measured by deaths ‘saved’ against those predicted by the unsubstantiated simulated forecasts of the modelling.
Aside from the reportedly dismal coding, was it robust? Models based on assumptions in the absence of data can be over-speculative and open to over-interpretation. Professor John Ioannidis of Stanford University issued a strong warning5 for disease modellers to recognise the deficiencies in reliable data about Covid-19, which in time proved to be transmission, fatality rates and T-cell reactivity (See Chapter 10, ‘The metrics of fear’, for more detail.)
The modelling also did not take take into account the spread of the virus in hospitals, care homes and prisons. When 40%6 of deaths are care home residents and up to two thirds of infections leading to serious illness are contracted in hospital,7 it cannot be over-stated
Comments (0)